I have been working in a software company for almost two years now. We provide data management solutions to a large range of clients, from fast-growing start-ups to global corporates. This is my first role in technology after my degree in Corporate Finance and working in finance roles in industrial corporate since then.
I have always felt at ease working with data and I consider myself skilled at crunching, reading and reporting them. In a rather naive fashion I thought of this as a natural transition.
What I did not realise until two years ago is that the data that I was exposed to as analyst are the final product of a supply chain that I wasn’t fully aware of. In a sense, I have used data without fully appreciating where they were coming from, or the effort that goes into producing high value, reliable data. In metaphorical terms I was like a chef that didn’t know the supply chain of the ingredients.
I believe I was not an exception in that context as the role of analysts is mainly to collect data from systems or spreadsheet and use them to produce reports. The main assumption here is that those data inputs are good, which in a corporate environment means believing that someone else is making sure that data are well collected, maintained, updated, consolidated, copied and even (!) periodically cleaned-up.
Data users vs. data workers
Finance analysts are data users. For them, data are tools used to support decision making. Sometimes external pressure and time constraints may induce finance departments to use them even when they are not as good as they should be. Loopholes and shortcuts in the process may put data quality at risk as any manual intervention on the data could contain human mistakes and inconsistencies.
In the world of data-as-tools, I focused significant care and attention on the outputs: clear charts, crisp reports and numbers that were adding up to the cent. The “art” of the analyst is to produce the best recommendations they can with tools at their disposal.
Data workers are those people that consider data-as-a-product and their role is to make sure that the final users receive data of the best quality possible.
In the world of data-as-a-product I’m becoming much more aware that the quality of the output is only as good as the quality of the input. In a sense, while using even imperfect data to generate reports and BI will likely serve the purpose of moving on decisions in the short run, in the medium-long term it could damage the organisation by providing polluted recommendations.
A shift in priorities
If you asked me few years ago about the problems affecting reporting functions in large corporations I would have talked about the impacts of operational changes on accounting. Quick changes in the organisation make it difficult for a support role like finance to keep up and maintain an acceptable level of quality in the reports.
What I have realised since delving deeper into the sources and flows of corporate data is that data quality is a widespread challenge and struggling to keep pace with changes is a by-product of poor data processes. Quality data is not just consistent, but also available, well-sourced, updated, comparable, credible and reliable.
Achieving this result is not an individual sport. Finance cannot solve the issue of bad data alone with inputs coming from sales, operations, HR and beyond.
Without efficient collection, collaboration and sharing of data, crucial information can be lost at every intermediate step to detriment of the quality of the final reporting.
Realisations
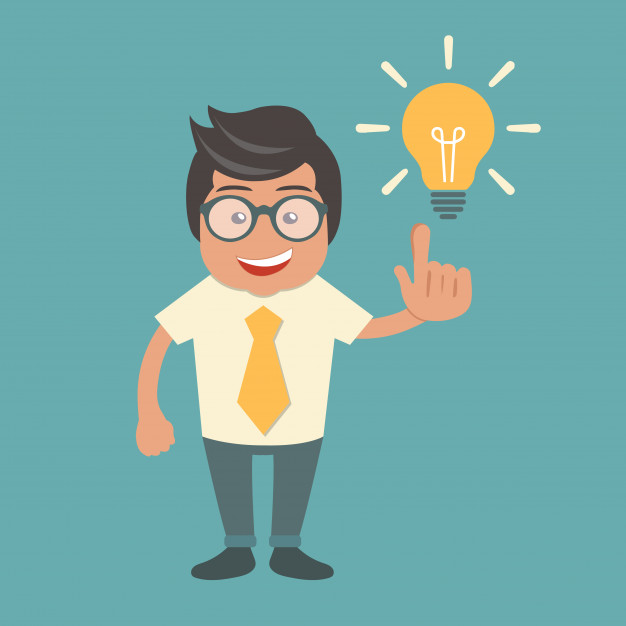
In my brief but revealing journey from a data user to data worker, I will try and summarise my key realisations in 5 bullets that I will expand further in subsequent posts:
- Cost of crunching: as an analyst I was spending 80% of my time reconciling, preparing and ‘crunching’ the data rather than analysing them.
- Cost of (poor) quality: the quality of a report is only as good as the quality of the underlying data. Poor quality data that is allowed to survive through the day-to-day can accumulate significant hidden costs for the organisation.
- Cost of inertia: for some companies, having solid data quality procedures in place is still seen as a nice-to-have, an investment that can be postponed until they have no choice but to act – faced with regulatory pressures or other external factors.
- Cost of time: data quality is not an intrinsic characteristic of data but it is influenced by several factors: distance from the source, availability, comparability and most importantly time. Ideally companies should try and shorten the distance between reporting functions and the field – either operations or sales or HR – in order to have a better snapshot of the reality.
- Cost of (poor) tools: for data users, spreadsheets will continue to be an invaluable tool for interrogating, manipulating and analysing data. And as a single-user tool that is fine but wherever you need to collaborate with more than a handful of other contributors in the organisation they just aren’t scalable and certainly cannot replace a system.
Being able to describe the problem is the first step
I spent the first years of my professional career reconciling information affected by technological constraints. During the high-pressure periods, we were all wishing for a smarter solution, but as the pressure lowered, triggers for investigation disappeared and we convinced ourselves that the status quo was acceptable.
A couple of years later, I find myself in a better position to articulate the issues affecting analyst roles than when I was one.
Now, it is clear to me that as soon as data quality concerns arise it is advisable to take a step back and assess the impact before the situation becomes unbearable. Implementing appropriate systems, re-shaping processes and engaging consultants is an investment in the future wealth of a company and the bigger the scale, the larger the savings.
Being able to describe the issues that affect your function can be the first step to finding a solution. And sometimes, it takes a little help from the outside to tease out the details.
Cover image and pics: freepik.com